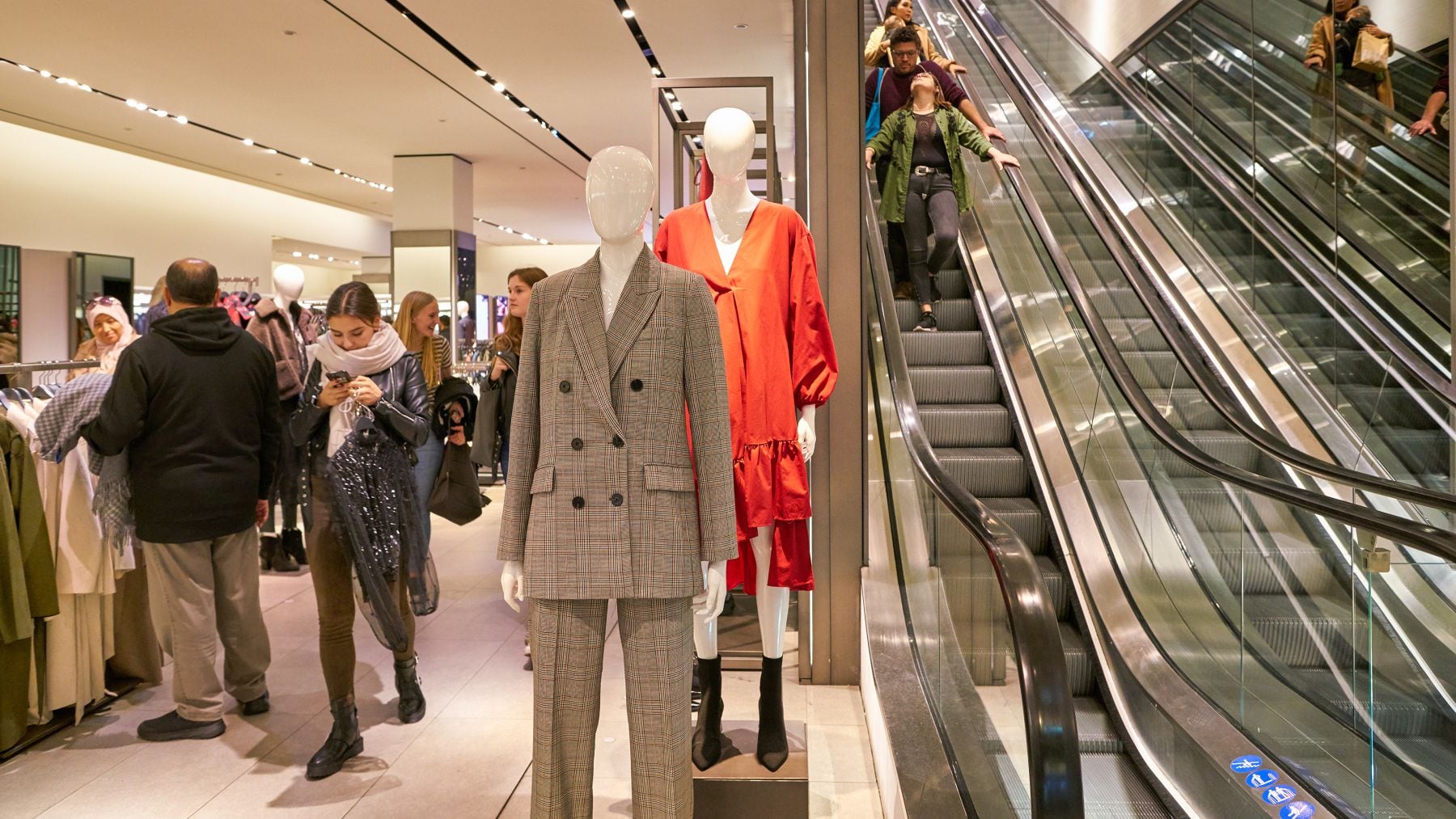
Of the services that Sparkbox, an AI-centric retail planning platform, offers to customers, one seeing demand uses AI to place quick in-season reorders of products that are performing well. Another gaining momentum leverages the technology to optimise pricing.
But one yet to find users is its AI-powered demand forecasting for initial buys, which predicts a product’s performance weeks into the future.
“It’s something that a lot of people are asking about, but it’s not something that anyone wants to immediately do,” said Sparkbox co-founder Lindsay Fisher. “No one wants to take the first step.”
Brands are still sceptical about the validity of AI forecasts, she said, and to make decisions with AI about their initial buys, they need to have clear, quantifiable assumptions to base their models on. Many, however, still rely on gut feeling.
Sophisticated AI forecasts are still a relatively new tool for fashion, and companies are still working out how much to rely on them. They’re also up against the fact that fashion purchases are often driven by emotion, novelty and the strength of the particular product, meaning previous sales, even of similar items, can only tell you so much.
Of course, human merchandisers have always had to predict the future when placing their initial production orders for all the clothes that will fill their stores and online shops. The average fashion business operates on lead times of 37 to 45 weeks, according to Boston Consulting Group. That means they’re guessing every style, colour and size customers will buy as far as 10 months in advance. Wrong guesses translate to money lost on discounted and unsold items, as well as more waste.
In theory, AI could offer more accurate forecasts than historical methods, which combine mathematical models applied to past sales, and maybe a few variables, with pure instinct. AI, by contrast, is able to take in far more data, on the order of dozens to hundreds of variables, finding unexpected relationships and signals that potentially allow for better predictions.
But not everyone believes it’s ready to fully take over the job of setting initial buys.
“If you’re trying to forecast the future, AI is not going to help you,” said Ahmed Zaidi, co-founder and chief executive of Hyran Technologies, an AI-powered planning and supply chain platform for fashion. “If anything, it’s probably more dangerous than the way we used to do it before, because it’s disguising itself as objective.”
The Limits of AI
What’s frequently left out of conversations about AI, according to Zaidi, is that it’s probabilistic. Forecasts should come with confidence scores, he said, since a prediction might only have 40 percent confidence. That doesn’t generally happen.
It can have other shortcomings as well. Researchers who conducted a recent survey of different forecasting methods noted that AI methods could be challenging to implement, require lots of high-quality data and be subject to idiosyncrasies like “overfitting,” where a model learns irrelevant data in its training and can’t make accurate generalisations from new data. It’s as if the system learned the performance of one red shirt so well that it couldn’t make reliable predictions about a new batch of similar shirts, for example.
The researchers didn’t directly compare the effectiveness of different methods, but any method at this stage is challenged to make accurate guesses about what shoppers will want several months down the road.
“With the initial-buy planning, there’s a lot more opinion involved, so maybe AI isn’t as useful in that respect,” said Alex Ure, head of merchandising for Jaded London, which scored a hit earlier in the year when Hailey Bieber wore a pair of its parachute pants at Coachella. “There’s always going to be that element of trend and [creative] strength that I don’t think an application would harness.”
Ure herself has sometimes pushed back on the wilder initial buys the creative team advocated for and turned out to be wrong, she said.
Jaded isn’t opposed to AI. It recently began using Sparkbox’s tools for reordering and is about to start testing its pricing optimisation. It might try AI-based forecasting for initial buys at some point, Ure added, but it’s not quite there yet.
AI’s Capabilities
AI doesn’t have to be perfect to be useful, though.
“It’s a fair point that if you suddenly feel that the science is going to do all the work and there is no art involved, you’re probably going too far in the wrong direction,” said Santiago Garcia-Poveda Maria, global vice president of retail, apparel and footwear at o9 Solutions, an end-to-end planning platform that offers AI-based forecasting to clients such as Marks & Spencer, J.Crew and several large sporting-goods brands.
Garcia-Poveda Maria said the company has seen several instances where AI forecasting is helping brands forecast more effectively, pointing to significant improvements at one US-based footwear brand it worked with compared to its initial planning.
“Either in an assisted way or in a fully automated way, I think clearly the human decision can be improved,” said Carlos Sánchez Altable, a partner and lead in data analytics for apparel, fashion and luxury at McKinsey.
In his experience, many companies are now using AI to help with their initial buys at the category level, but it’s still rare for companies to do it at the level of size and colour. Though he believes many will eventually adopt it for that use, too.
There are numerous ways companies can use AI for forecasting outside of initial buys as well, he noted. One example would be reserving ironing capacity in a logistics centre. When products arrive at European facilities from factories in Asia, they often need ironing before they can be displayed or sold. Companies have to reserve that capacity.
Similarly, when o9 Solutions is dealing with a brand focused on new, trendy products, it doesn’t try to say 12 months in advance exactly how many units in each colour and size they’ll need. It focuses on gauging how much capacity the brand should reserve with its factory partners. That way, when the company is ready to go into production, it has the time and materials already reserved, allowing it to move faster.
Optimising, as opposed to predicting, is where AI shines, in Zaidi’s view. These uses are more along the lines of deciding which products to allocate to which stores, or determining the best production decision amid a number of different scenarios — the specialty of Hyran Technologies. The technology is a powerful tool for allowing brands to constantly update the scenarios they’re basing their forecasts on, which is less about predicting demand and more about letting brands quickly adjust to new information as it arises.
Operating that way requires flexibility in the supply chain, and that remains a key priority for countless companies. The shorter the lead time, the better it’s able to respond to actual shopper behaviour rather than far-off predictions.
Though at some point, someone — or something — will need to make a decision.
“It’s the same problem that we always discuss,” Sánchez Altable said. “The human, the art, the science — how do you put that together?”
Editor’s Note: This story was amended on Dec. 12, 2023, to clarify that Sparkbox’s forecasting for initial buys has yet to find users.